KEY TAKEAWAYS
- Generative AI models can be used to violate privacy. Instead of being afraid of such language models, policymakers must develop policies and regulations that strike a balance between protecting data privacy and promoting innovation.
- These models may inherit the biases present in the data it is trained on which could lead to discrimination against individuals based on their identity. However, diversifying training data, fairness constraints, adversarial training, and counterfactual data augmentation will mitigate bias in generative models.
- Language models can be used for cyberattacks and deep fakes.
- In the context of Bangladesh, unethical uses of generative models could lead to exacerbating existing social tensions or fueling political instability through fake news or propaganda generated by language models.
- Ethical uses could revamp the personalized healthcare ecosystem, bridge communication barriers, and promote cross-cultural understanding.
- Measures such as antitrust regulations and investments in workforce training and education will help ensure to share the benefits of AI language models more equitably.
1. Background
In this section we will discuss the relevant background concepts of ChatGPT and other generative AI Models. First we describe what is a generative AI model.
1.1 Generative AI Models
Generative AI models are machine learning models that can provide textual [11, 41] or image [36, 33,27, 37], video [22, 39, 15], audio [43, 14, 8, 28] outputs from text or multimodal prompts [3]. The basic principle of generative model is quite simple. Every specific image have some featurs or some properties associated with it. There are certain properties that make a four legged animal a dog. If we want to generate a picture of a dog, we first need to learn what makes a dog a dog – things like the shape of their ears, puppy eyes, the color of their fur, and the shape of their body etc. Once the model has learned these patterns, we can ask it to generate a new image of a dog. The model first learns the pattern and then generates new dog that might look realistic enough but something that never existed in real life. We can consider these generative models in a similar way as human babies learn. Babies observe their environment, recognize patterns, take feedback from parents and environments and finally use all of these to make learn patterns of which furry animals are dogs. Armed with this knowledge, human babies can eventually identify which animal is a dog or which dog is their dog and so on. But existing generative models require a huge amount of data to learn patterns of a dog compared to a human baby, so there are some differences also.
As we see the current trend of large language models, we have used even 1 Trillion parameters in models like GLaM as shown in figure 1 Almost all the tech giants like Google, Facebook, Microsoft, OpenAI have published their recent large language models very recently. The pace of this development is so fast, that even the most modern nations in the world are not capable of keeping up with it. In the United States, policies like section 230 from the Communication Decency Act 1996 that protected social media sites, is not capable enough to deal with it. It strictly separated content delivery from content creation. But generative models are increasingly blurring that boundaries.
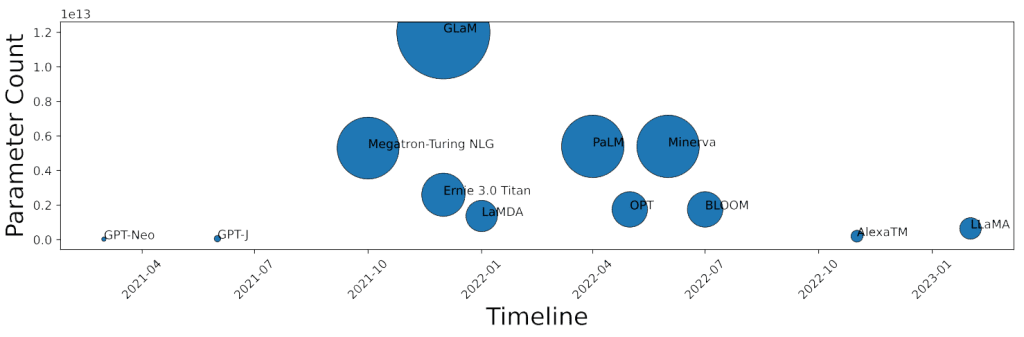
1.2 ChatGPT
ChatGPT itself is a product launched by OpenAI. The underlying architecture is based on the GPT-3 model. However due to ease of use, availability, functionality and many other fascinating properties, ChatGPT brought out revolution in AI based product uses. It became one of fastest products if not the fastest ever that was launched online, to reach 1 million users as shown in Figure 2 What sets OpenAI’s ChatGPT from other model is it’s usability and ease of access. Underlying details of the model is not shared publicly, however, from the press release and discussion, we can understand that this model is also based on GPT-3 [11] which had similar result, but the interface was not popular. When ChatGPT was released, it reached 1 million user mark within 5 days only. For comparison, Netflix took 3 and half years to reach one million users.
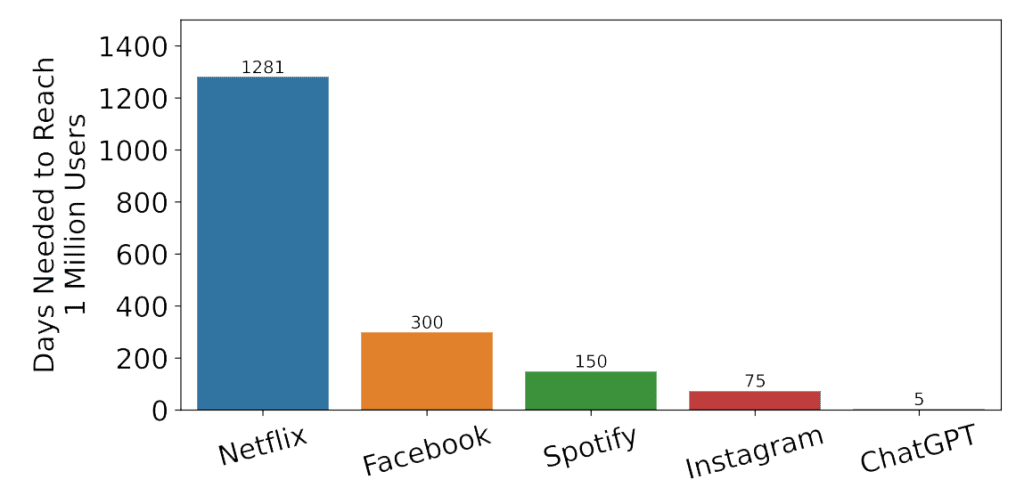
ChatGPT from other model is it’s usability and ease of access. Underlying details of the model is not shared publicly, however, from the press release and discussion, we can understand that this model is also based on GPT-3 [11] which had similar result, but the interface was not popular. When ChatGPT was released, it reached 1 million user mark within 5 days only. For comparison, Netflix took 3 and half years to reach one million users.
ChatGPT, like most of the Generative Pre Trained (GPT) models, were trained on large scale of data with billions of words from movies, books, news articles and websites. This data was used to capture different patterns and intricacies of the natural language. These datasets include – BookCorpus [45], English Wikipedia and WebText [32] dataset. As ChatGPT is trained on this huge dataset, it can deliver the contents quite surprisingly well. Recently, the upgraded version of ChatGPT, namely GPT-4 [29] has shown further capabilities of passing different real life and challenging examinations like Uniform Bar Exam in 90th percentile, SAT EBRW at 93rd percentile, SAT Math at 89th percentile, GRE Quant at 80th percentile, GRE Verbal at 99th percentile.
Since it’s inception, ChatGPT has been used in many unique cases from assisting in giving verdict in a First Circuit Court [1], negotiating with lawyers [23], writing boooks [34], writing scientific articles [18] In many cases, backlashes followed on due to not having enough policy measures.
2. Data Privacy
Privacy is often considered as a human rights [4]. However, due to the data dependent method of machine learning models, we have seen data privacy to become the foremost concern in most of the Generative AI Models. There has been numerous attack methods discussed that can be used to breach the privacy of machine learning models [25, 38, 12, 42].
Language models like ChatGPT require large amounts of data to train, and this data often contains personal information that may be sensitive or private. This data can include text messages, emails, social media posts, and other forms of online communication. If this data falls into the wrong hands, it could be used for malicious purposes such as identity theft or phishing scams.
For example, consider a hypothetical scenario where a company uses a language model to generate personalized emails for its customers. The model is trained on the company’s customer database, which includes names, addresses, and email addresses. If the data is not properly secured, it could be vulnerable to cyberattacks, potentially exposing sensitive customer information to malicious actors.
In addition to the risk of data breaches, there is also the risk of unintentionally revealing personal information through the language model’s outputs. For example, if the model is trained on a dataset that includes personal information such as race, gender, or age, it could inadvertently reveal this information in its outputs, potentially leading to discrimination or other harms.
While policymakers should be aware of these data privacy concerns, they should not necessarily be afraid of language models like ChatGPT. Instead, they should work to develop policies and regulations that address these concerns while still allowing for the development and deployment of these technologies. This could include measures such as data protection regulations, cybersecurity standards, and ethical guidelines for the use of language models.
Ultimately, policymakers need to strike a balance between protecting data privacy and promoting innovation and development in the field of artificial intelligence. By doing so, they can help ensure that these technologies are developed and used in a responsible and ethical manner.
3. Bias & Fairness
Generative AI models may inherit the biases present in the data it is trained on. This could lead to discrimination and unfair treatment of individuals based on factors such as race, gender, or socioeconomic status. We have seen this in applications like Correctional Offender Management Profiling for Alternative Sanction COMPAS [7] that had significant bias based on the race of the offender. It is crucial to evaluate and mitigate these biases to ensure that ChatGPT’s outputs are fair and equitable.
Generative models like ChatGPT are susceptible to bias in their outputs, as they learn from patterns in the data they are trained on, and this data can reflect societal biases and stereotypes. This can lead to discriminatory or unfair outcomes in the model’s outputs. We have previously seen chatbots like Tay [44, 26] have faced tragic end. It took less than 24 hours for the Twitter users to corrupt the AI chatbot. Microsoft hurriedly killed this service. Similar fate can await for any system that would not preemptively try to fight against such biases in the training dataset.
There are several techniques that can be used to mitigate bias in generative models, including:
1. Diverse Training Data: Using a more diverse range of training data can help ensure that the model is exposed to a broader range of perspectives and experiences, reducing the risk of bias in its outputs. One approach to diversifying the training data for generative models like ChatGPT is to increase the representation of underrepresented groups in the dataset [17]. This can be done by collecting more data from diverse sources or by using techniques like data augmentation to increase the diversity of the existing data.
2. Fairness Constraints: Researchers can use mathematical constraints to ensure that the model’s outputs do not discriminate against specific groups [20]. For example, they can add constraints that require the model to produce outputs that are statistically similar across different demo-graphic groups. These methods have been used to mitigate unintended biases in the Cyberbullying detection models [13]
3. Adversarial Training: This technique involves training the model to be robust against attacks that attempt to introduce bias into its outputs. Techniques like debiasing and adversarial training to reduce bias in the model [2] has been employed in production. Debiasing involves modifying the training process or the model architecture to reduce the impact of biases in the data.
Suppose we have a generative model that is trained on a dataset of movie reviews. We want to ensure that the model doesn’t generate biased reviews that unfairly favor certain groups of people, such as men or women. To do this, we can use adversarial training to train the model to recognize and correct for gender bias in the data. Specifically, we can create adversarial examples by swapping the genders of characters in the movie reviews, so that a review that originally referred to a male character now refers to a female character, and vice versa. These adversarial examples are designed to trick the model into generating reviews that are gender-neutral, rather than biased toward one gender or the other.
We then train the model on a combination of the original movie review dataset and the adversarial examples. During training, the model learns to recognize and correct for gender bias by trying to distinguish between the original examples and the adversarial examples. As a result, the model becomes more robust to gender bias and is able to generate reviews that are fairer and more inclusive.
4. Counterfactual Data Augmentation: This involves adding counterfactual examples to the training data, which are examples that introduce a small change to the input data to make it less biased. Suppose we have a dataset of job applications, and we want to train a model to predict which applicants will be successful. However, the dataset contains biases, such as gender bias, that could lead to unfair predictions.
To address this issue, we can use counterfactual data augmentation to generate new data points that represent counterfactual scenarios, such as what would happen if the gender of an applicant were different. Specifically, we can take each original data point and modify it in a way that alters the outcome, while keeping other features the same. For example, we can change the gender of a female applicant to male, and vice versa.
We can then use these counterfactual data points to train the model, in addition to the original data. By training on the counterfactual data, the model can learn to recognize and correct for biases in the original data, and make fairer predictions. This has been verified to be effective in real-world dataset in multiple studies [19, 40]
5. Post-processing Techniques: Currently, in many cases, ChatGPT or similar model produces an output stating it’s limitation as a large language model. However, these are triggered by many prompts which can actually be processed. This has been validated by the fact that there were nu-merous attempts to jailbreak ChatGPT and spill out outputs which are otherwise not allowed [16]. However, without this jailbreak scenarios, ChatGPT use post-processing techniques to remove any biases that may be present.
Overall, addressing bias in generative models is an ongoing area of research, and there is no one-size-fits-all solution. It is important to continue developing and testing new techniques to ensure that these models are fair and equitable.
4. Ethical Usage
Any generative model can be used for a variety of applications, including chatbots, customer service, and even creating fake news or deepfakes. It is essential to establish ethical guidelines for its use to prevent harm and misuse.
We have to be aware of the following unethical usecases where the power of the generative models can be exploited –
1. Cyberattacks: Generative models can be used to generate realistic phishing emails or other social engineering attacks, which can be used to steal sensitive information or money from unsuspecting victims. Good thing is OpenAI have deployed a red team to test whether their newer models like GPT-4 [29] can be used to do targeted cyberattacks or not. Based on this tests, they deemed the model safe to be released to public.
2. Deepfakes: Generative models can be used to create realistic deepfake videos, which can be used to spread false information or defame individuals. There had been cases of using Deepfake technology being used to spread conspiracy theories before social media websites or internet monitoring sites could do anything. [6]. There have been numerous research attempts to correctly identify such deepfake videos. For example, one study used the rate of blinking in videos to classify them as deepfakes or not [21]. However, as the generative models get better, it gets more difficult to separate deepfake videos from other authentic ones. At this point, it becomes more of a cat and mouse games between deepfake creators vs detectors. Another problem fighting against the deepfakes are implementing such technologies across the regular websites and social media. For example, social media sites have trouble implementing their existing policies against hateful speeches [24]. so, implementing even more complicated policies to detect deepfake would be another hurdle to overcome.
In the context of Bangladesh or other least developed countries, unethical uses of generative models could be particularly harmful. For example, fake news or propaganda generated by a language model could exacerbate existing social tensions or fuel political instability. Similarly, cyberattacks or deepfakes generated by a model could have serious consequences for individuals or organizations with limited resources to recover from such attacks.
On the other hand, ethical uses of generative models could have significant benefits for least developed countries. For example, personalized healthcare recommendations generated by a language model could help improve health outcomes in areas with limited access to medical professionals. Similarly, language translation generated by a model could help bridge communication barriers and promote cross-cultural understanding.
5. Legal Responsibilties
As ChatGPT or similar generative models can generate outputs that mimic human language, there may be questions of legal responsibility and liability for its actions. It is crucial to establish clear legal frameworks to address these issues. Determining responsibility for the unethical use of generative models like ChatGPT can be complex and depend on the specific circumstances of each case. However, it is important to recognize that both the user and the creators of the technology can have a role to play in ensuring responsible and ethical use. In general, the user of a generative model who deploys it for unethical purposes would be primarily responsible for any harm caused. Currently, there are no specific laws in USA that govern the policies of generative AI models. Whether Section 230 is applicable or not that is also highly debated. Other nations are also struggling to decide on whether they would want to implement newer policies governing the outcomes of generative AI models.
This is similar to how individuals who commit crimes using other forms of technology, such as computers or smartphones, are held accountable for their actions. However, the creators of the technology could also potentially share some responsibility if they failed to take appropriate steps to prevent or mitigate unethical uses of their product. For example, if we recall the case of Stable Diffusion, the CEO of the company that launched this product, mentioned that any user can download the model in their own computer and then train as they wish with whatever dataset while they were being subject to online discussion regarding the violent imagery [10]. The CEO also shared GPU lists which are compatible to run those models. This sort of behavior encourages usecases which are harmful for the overall society.
To help mitigate the risks of unethical use of generative models, companies like OpenAI or other creators of such models can take several steps, including:
Developing and Enforcing Ethical Guidelines: Companies can create guidelines and policies for the responsible use of their products and enforce them through user agreements or other means. For example, OpenAI has established ethical principles for its research and development activities. GDPR [35] can be a starting point for such frameworks.
Implementing Technical Safeguards: Companies can incorporate technical safeguards into their products to prevent or mitigate the risks of unethical use. For example, some companies have developed algorithms to detect deepfakes or other forms of synthetic media. Popular gif hosting platform like Gyfcat have algorithms to analyze frame by frame content for detecting deepfakes [30]. Popular video sharing platform YouTube uses combination of machine learning, human review as well as user reports to optimally detect deepfake videos.
Collaborating with Experts: Companies can work with experts in relevant fields, such as computer ethics, media studies, or law enforcement, to develop strategies for addressing ethical challenges and potential misuse of their products.
Ultimately, it is important for companies that create generative models and other forms of advanced technology to take responsibility for the potential risks associated with their products and to work to ensure that they are used in a responsible and ethical manner.
6. Economic Concerns
Large language models have been found to have significant economic impacts. The development and deployment of such models have implications for employment, income distribution, and market competition. For instance, AI language models can automate tasks that were previously performed by humans, leading to job losses in some industries and sectors. At the same time, the use of AI models can create new job opportunities in fields such as data science and AI engineering [5].
On their recent paper with University of Pennsylvania researchers, OpenAI team have found that many jobs will be impacted by GPT models [9]. They have showed that jobs like Tax Prepares, mathematicians, writers, authors, accountants, news analysts will have higher chances of getting replaced by AI. According to Harris Poll, 40% of workers who are familiar with ChatGPT thinks that AI will eventually replace their jobs. However, some 60% are optimist that generative AI model will make them more productive.
In addition, large language models are often proprietary and owned by big tech companies, leading to concerns about market competition and monopolies. The dominance of a few companies in the AI industry can limit innovation and raise barriers to entry for smaller companies.
To address these concerns, policymakers can consider measures such as antitrust regulations and investments in workforce training and education [31]. These efforts can help ensure that the benefits of AI language models are shared more equitably and that the overall impact on the economy is positive.
About the Author
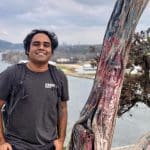
Farabi Mahmud is currently doing his PhD in Computer Science and Engineering at Texas A&M University. A former debater, he is the head of Youth Policy Forum’s Technology and Digital Engagement.
References
[1] Judge used chatgpt to make court decision. https://www.vice.com/en/article/k7bdmv/judge-usedchatgpt-to-make-court-decision, 2022. Accessed: March 25, 2023.
[2] Bai, X., Guan, J., and Wang, H. A model-based reinforcement learning with adversarial training
for online recommendation. Advances in Neural Information Processing Systems 32 (2019).
[3] Bang, Y., Cahyawijaya, S., Lee, N., Dai, W., Su, D., Wilie, B., Lovenia, H., Ji, Z., Yu, T., Chung, W., et al. A multitask, multilingual, multimodal evaluation of chatgpt on reasoning, hallucination, and interactivity. arXiv preprint arXiv:2302.04023 (2023).
[4] Banisar, D. Privacy and Human Rights…: An International Survey of Privacy Laws and Developments. Electronic Privacy Information Center, 1999.
[5] Bessen, J. Automation and jobs: When technology boosts employment. Economic Policy 34, 100 (2019), 589–626.
[6] Brown, N. I. Deepfakes and the weaponization of disinformation. Va. JL & Tech. 23 (2020), 1.
[7] Chouldechova, A. Fair prediction with disparate impact: A study of bias in recidivism prediction instruments. Big data 5, 2 (2017), 153–163.
[8] Dhariwal, P., Jun, H., Payne, C., Kim, J. W., Radford, A., and Sutskever, I. Jukebox: A generative model for music. arXiv preprint arXiv:2005.00341 (2020).
[9] Eloundou, T., Manning, S., Mishkin, P., and Rock, D. Gpts are gpts: An early look at the labor market impact potential of large language models, 2023.
[10] Eshoo, A. Eshoo urges NSA, OSTP to address unsafe AI practices. https://eshoo.house.gov/media/press-releases/eshoo-urges-nsa-ostp-address-unsafe-ai-practices, June 2022. [Online; accessed 25-March-2023].
[11] Floridi, L., and Chiriatti, M. Gpt-3: Its nature, scope, limits, and consequences. Minds and Machines 30 (2020), 681–694.
[12] Fredrikson, M., Jha, S., and Ristenpart, T. Model inversion attacks that exploit confidence information and basic countermeasures. In Proceedings of the 22nd ACM SIGSAC conference on computer and communications security (2015), pp. 1322–1333.
[13] Gencoglu, O. Cyberbullying detection with fairness constraints. IEEE Internet Computing 25, 1 (2020), 20–29.
[14] Hadjeres, G., Pachet, F., and Nielsen, F. Deepbach: a steerable model for bach chorales generation. In International Conference on Machine Learning (2017), PMLR, pp. 1362–1371.
[15] Hu, Y., Luo, C., and Chen, Z. Make it move: Controllable image-to-video generation with text descriptions. In Proceedings of the IEEE/CVF Conference on Computer Vision and Pattern Recognition (2022), pp. 18219–18228.
[16] Jenkins, M. Chatgpt ’alter-ego’ dan: Users jailbreak ai program to get around ethical safeguards. The Guardian (March 2023).
[17] Kamiran, F., and Calders, T. Classification with no discrimination by preferential sampling. In Proc. 19th Machine Learning Conf. Belgium and The Netherlands (2010), vol. 1, Citeseer.
[18] King, M. R., and chatGPT. A conversation on artificial intelligence, chatbots, and plagiarism in higher education. Cellular and Molecular Bioengineering (2023), 1–2.
[19] Kusner, M. J., Loftus, J., Russell, C., and Silva, R. Counterfactual fairness. Advances in
neural information processing systems 30 (2017).
[20] Li, P., Zhao, H., and Liu, H. Deep fair clustering for visual learning. In Proceedings of the IEEE/CVF Conference on Computer Vision and Pattern Recognition (2020), pp. 9070–9079.
[21] Li, Y., Chang, M.-C., and Lyu, S. In ictu oculi: Exposing ai generated fake face videos by detecting eye blinking. arXiv preprint arXiv:1806.02877 (2018).
[22] Li, Y., Min, M., Shen, D., Carlson, D., and Carin, L. Video generation from text. In
Proceedings of the AAAI conference on artificial intelligence (2018), vol. 32.
[23] Lopez, N. Donotpay now negotiates your bills with an ai chatbot. The Verge (December 13 2022). Accessed: March 25, 2023.
[24] Manjoo, F. Twitter, free speech and the truth. The New York Times (August 2018). Accessed: March 26, 2023.
[25] Melis, L., Song, C., De Cristofaro, E., and Shmatikov, V. Exploiting unintended feature
leakage in collaborative learning. In 2019 IEEE symposium on security and privacy (SP) (2019), IEEE, pp. 691–706.
[26] Neff, G. Talking to bots: Symbiotic agency and the case of tay. International Journal of Communication (2016).
[27] Nichol, A., Dhariwal, P., Ramesh, A., Shyam, P., Mishkin, P., McGrew, B., Sutskever,
I., and Chen, M. Glide: Towards photorealistic image generation and editing with text-guided
diffusion models. arXiv preprint arXiv:2112.10741 (2021).
[28] Oord, A. v. d., Dieleman, S., Zen, H., Simonyan, K., Vinyals, O., Graves, A., Kalchbrenner, N., Senior, A., and Kavukcuoglu, K. Wavenet: A generative model for raw audio.
arXiv preprint arXiv:1609.03499 (2016).
[29] OpenAI. Gpt-4 technical report, 2023.
[30] O’Brien, S. A. Deepfakes are coming. is big tech ready. CNN Business (2018).
[31] Petit, N. Antitrust and artificial intelligence: a research agenda. Journal of European Competition Law & Practice 8, 6 (2017), 361–362.
[32] Radford, A., Wang, J., Amodei, D., and Sutskever, I. Webtext dataset. https://einstein.ai/research/webtext/, 2019. Accessed: March 25, 2023.
[33] Ramesh, A., Dhariwal, P., Nichol, A., Chu, C., and Chen, M. Hierarchical text-conditional
image generation with clip latents. arXiv preprint arXiv:2204.06125 (2022).
[34] Reuters. ChatGPT launches ’boom’ ai-written e-books on amazon. Reuters (February 21 2023). Accessed: March 25, 2023.
[35] Rochel, J. Ethics in the gdpr: A blueprint for applied legal theory. International Data Privacy Law 11, 2 (2021), 209–223.
[36] Rombach, R., Blattmann, A., Lorenz, D., Esser, P., and Ommer, B. High-resolution image
synthesis with latent diffusion models. In Proceedings of the IEEE/CVF Conference on Computer Vision and Pattern Recognition (2022), pp. 10684–10695.
[37] Saharia, C., Chan, W., Saxena, S., Li, L., Whang, J., Denton, E. L., Ghasemipour, K.,
Gontijo Lopes, R., Karagol Ayan, B., Salimans, T., et al. Photorealistic text-to-image
diffusion models with deep language understanding. Advances in Neural Information Processing Systems 35 (2022), 36479–36494.
[38] Shokri, R., Stronati, M., Song, C., and Shmatikov, V. Membership inference attacks against machine learning models. In 2017 IEEE symposium on security and privacy (SP) (2017), IEEE, pp. 3–18.
[39] Singer, U., Polyak, A., Hayes, T., Yin, X., An, J., Zhang, S., Hu, Q., Yang, H., Ashual,
O., Gafni, O., et al. Make-a-video: Text-to-video generation without text-video data. arXiv preprint arXiv:2209.14792 (2022).
[40] Sun, T., Gaut, A., Tang, S., Huang, Y., ElSherief, M., Zhao, J., Mirza, D., Belding,
E., Chang, K.-W., and Wang, W. Y. Mitigating gender bias in natural language processing:
Literature review. arXiv preprint arXiv:1906.08976 (2019).
[41] Thoppilan, R., De Freitas, D., Hall, J., Shazeer, N., Kulshreshtha, A., Cheng, H.-T.,
Jin, A., Bos, T., Baker, L., Du, Y., et al. Lamda: Language models for dialog applications.
arXiv preprint arXiv:2201.08239 (2022).
[42] Tramer, F., Zhang, F., Juels, A., Reiter, M. K., and Ristenpart, T. ` Stealing machine
learning models via prediction apis. In USENIX security symposium (2016), vol. 16, pp. 601–618.
[43] Vasquez, S., and Lewis, M. Melnet: A generative model for audio in the frequency domain. arXiv preprint arXiv:1906.01083 (2019).
[44] Wolf, M. J., Miller, K., and Grodzinsky, F. S. Why we should have seen that coming:
comments on microsoft’s tay” experiment,” and wider implications. Acm Sigcas Computers and Society 47, 3 (2017), 54–64.
[45] Zhu, Y., Kiros, R., Zemel, R., Salakhutdinov, R., Urtasun, R., Torralba, A., and Fidler, S. Aligning books and movies: Towards story-like visual explanations by watching movies and reading books. In Proceedings of the IEEE international conference on computer vision (2015), pp. 19–27.